An Introduction to Deepfake

Deepfake is a fascinating technology with both good and dangerous use cases. Whatever aspects will be dominating, it will likely become a big hype and demonstrates the importance to regulate AI.
Image Corrections and Special Effects
Manipulation of images and videos exists since analog film processing. Simple image corrections such darken or lighten of image areas were applied in the traditional darkroom. Later, those techniques got replicated digitally in software applications like Photoshop together with much more comprehensive features to alter image pixels. The expression that images are "photoshopped" is a statement that images have been manipulated.
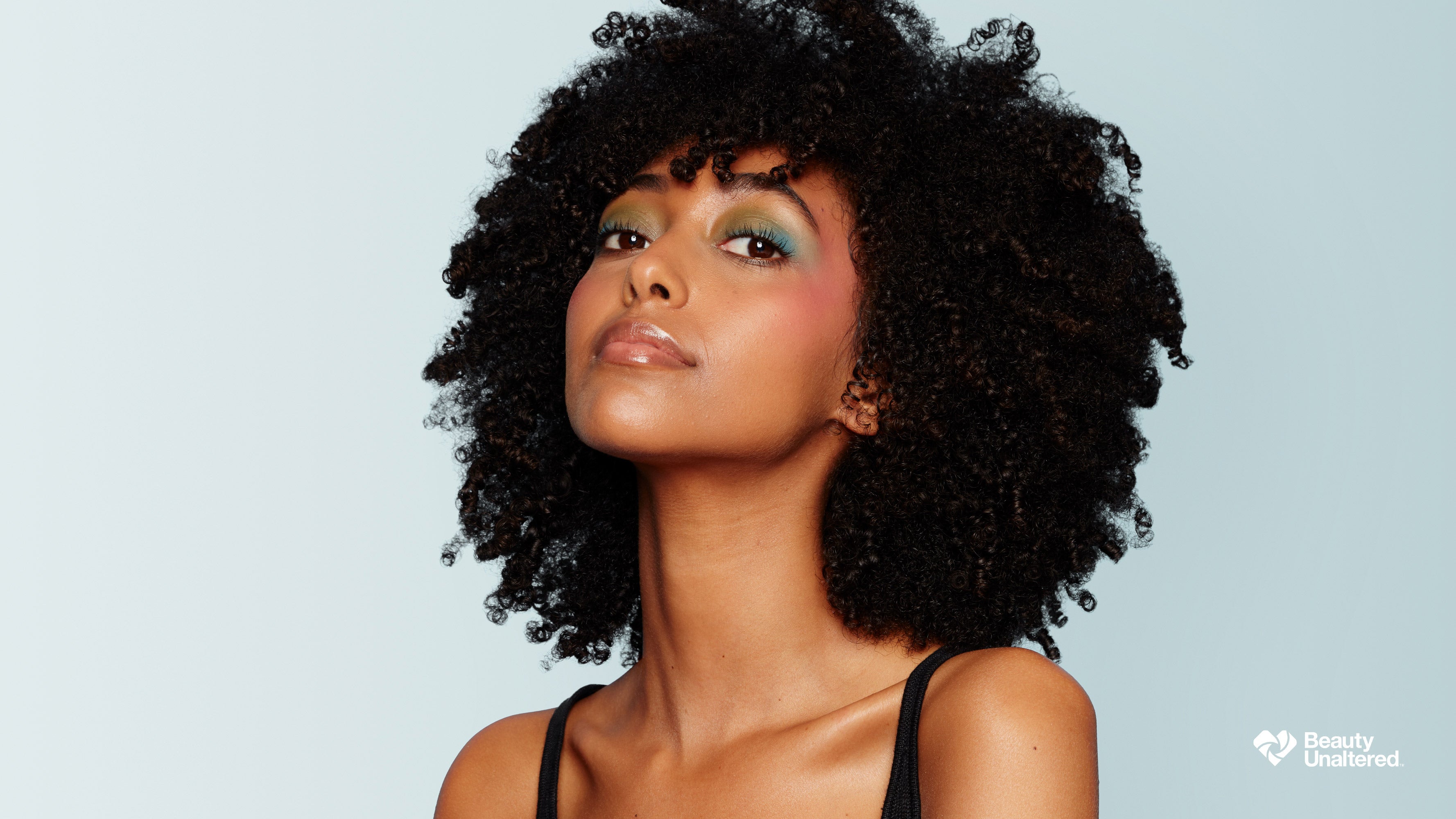
Equivalents in film and video production are special effects (SFX). Early approaches involved matte painting techniques to add painted image content to existing film scenes. The digital era introduced compositing software such as After Effects that has been recognized as a Photoshop for video (both by Adobe).
Typical Hollywood movies show a long list of SFX artists in the closing credits even if many tasks such as rotoscoping have been automated to large extent. Rotoscoping is a method to extract objects from a scene or remove backgrounds from foregrounds. Chromakeying (aka bluescreening or greenscreening in blue and green boxes) is a partly automated process of rotoscoping where green or blue backgrounds are replaced with different image content.
Video Conferencing
Using virtual backgrounds in video conferencing became very popular. Most people use virtual backgrounds to replace their work and home environments without leveraging the advantages of blue or green boxes. In this case, video conferencing applications try recognizing the person in front of the camera as foreground and extract them from what the software algorithm interprets as background. Results are not as precise as chromakeying but can be impressively good considering the low resolution of webcams in combination with poor light conditions.
Current implementations in video conferencing are based on fixed algorithms. Microsoft claims that their Teams video conferencing solution "uses a highly trained model" that differentiates users from backgrounds. This is a reference to an algorithm that got trained by Microsoft leveraging machine learning with sample content. At this point, it's fair to assume that no autonomously learning happens over time to personalize the experience around individual users in front of the camera and their backgrounds. The method could be further improved with deep learning but it would raise privacy concerns if the algorithm would process individual user data.

Deep Learning
To better understand how AI based video manipulation works, we need to first take a closer look on the different methods available within the spectrum of AI.
As the name suggests, deepfake is related to deep learning. In deep learning, software algorithms process unstructured data autonomously. Processing large amounts of image data is very suitable for deep learning to improve results independently from human interaction - except modifications of the core algorithm to allow developers improving methods of self-learning. Deep learning tries mimicking the neuronic learning process of the human brain. Like with humans, results become better with more practice. Developers are the teachers who introduce better methods but the learning process happens autonomously.
Deep learning is a subset of machine learning. A main difference is that machine learning typically processes structured data with predefined rules. Those rules are set by a human operator, which is called «rule based expert system». Machine learning runs within the boundaries of those rule sets. The concept of deep learning is to mimic human decision making allowing the rule based expert system to be replaced by deep learning's own logic.

Deep learning is a subset of machine learning and machine learning is a subset of artificial intelligence. AI is the much bigger buzzword than ML or DL. To attract investors or impress on a party, it's typically enough to highlight AI and not get into the geeky details.
Real Deepfake
Fakes are not supposed to be anything real but this classification doesn't apply here. From a pure technical point of view, a real deepfake is a deep learning based audiovisual manipulation.
Another aspect is that deepfakes don't need to be necessarily malicious. Deepfake methods can be leveraged to optimize video quality or improve video effects. Deepfakes can be a real looking and acting representatives of true people who want to be replaced by better appearances of themselves. A deepfake can be a realistic avatar of the same person that uses its own avatar. A camera captures facial expressions, body language and voice but the rendered output either replaces the captured video with a complete deepfake avatar or optimizes parts that aren't as good looking (or sounding). Would this be a fake or just image correction?
Looking at Zoom video conferencing, the "Touch up my appearance" option gives the picture a softer focus. It's literally photoshopping on-the-fly to make users look better, younger, fresher in the video call.

What if this were not just ordinary video filters with fixed values but instead deep learning based algorithms? Deep learning would get to know users over time, analyze their expressions and actings, compare with thousands and millions of other users, learn from comprehensive data. The optimized results would be astonishing and we assume that many people would want such effects to pimp their own appearance. It could also help in cases where a normal camera feed would fail, such as low-light situations where the sensor would produce grainy images or low bandwidth cause blocky artifacts.
What is fake and what is optimization? Before judging, let's keep freedom of expression in mind. We recommend listening to the below linked podcast by fxguide with Dr. Andrew Glassner, author of the book «Deep Learning: A Visual Approach». Towards the end of the podcast, the discussion touches on the controversial aspects.
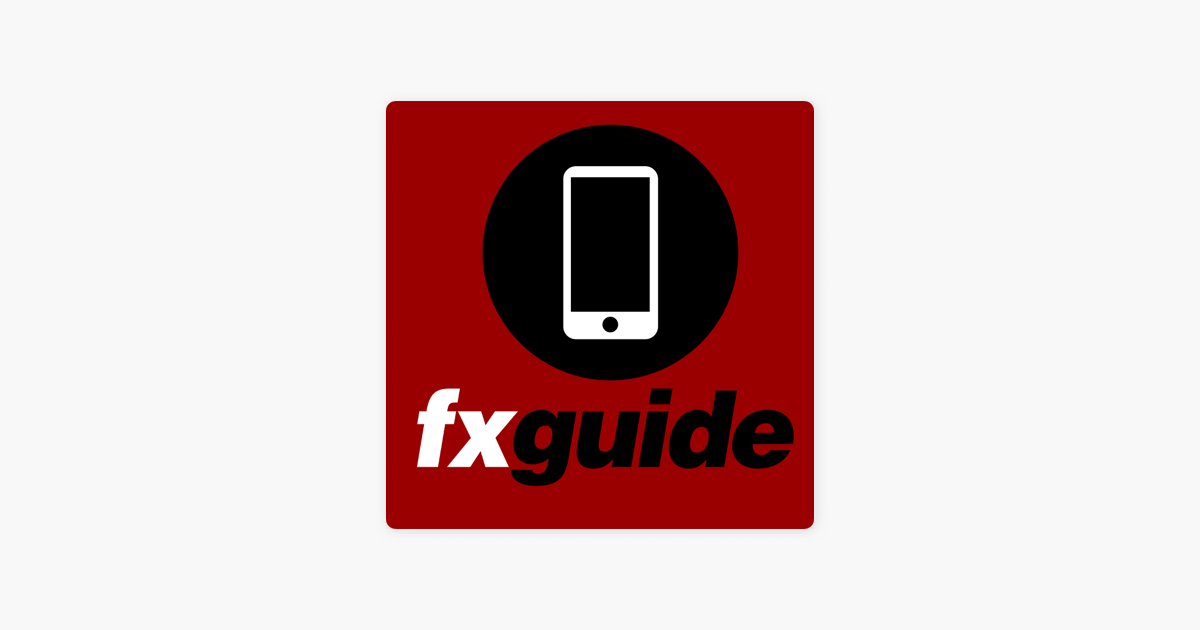
One-Click Deepfake Video
When we wrote the first version of this article in 2021, it was still required to feed deep learning tools with lots of data. The more input data in good quality, the better. Powerful PC workstations were required to process deepfakes within acceptable time. Often enough, the results were still not be perfect. To get close to Hollywood standards, some manual compositing was required in applications like After Effects. Voice deepfakes were a different beast. They often sounded electronically and unnaturally. Voice imitators could create better results in less time.
/cdn.vox-cdn.com/uploads/chorus_asset/file/10667925/maxresdefault.jpg)
Obama deepfake by Jordan Peele from 2018
Two years later, deepfake technology has evolved rapidly. Realstic deepfakes can be created in real time even on mobile devices. TikTok released a hyperrealistic beauty filter called Bold Glamour that demonstrated how easy and broadly AI based image manipulation was made available on smartphones to millions of users. The same rapid development took place with voice deepfakes.
/cdn.vox-cdn.com/uploads/chorus_asset/file/24473324/Bold_Glamor_TikTok_Filter_Effect.jpg)
TikTok’s filter runs on a mobile device, accessible by billions of people
AI vs. AI
Providers of deepfake detection solutions also leverage AI to identify deepfakes and media manipulation. However, it's hard to keep up because AI based deepfake detections are being trained with available deepfake data. It's a cat and mice game.
Vgency believes that AI algorithms for media manipulation need to be made available by law as open source. Proprietary, closed source based AI algorithms, its IP and patents need to be declared illegal and invalid. This would allow a broader community to control impact and to keep up with solutions for deepfake detection.
This blog post covers mostly visual deepfake. Read also our article about why voice deepfake is even more impactful.
